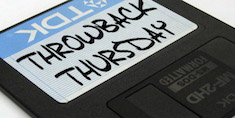
Crossing the Development to Production Divide
In this post we’ll give an overview of obstacles we’ve faced (you may be able to relate) and talk about solutions to overcome these obstacles.
In this post we’ll give an overview of obstacles we’ve faced (you may be able to relate) and talk about solutions to overcome these obstacles.
As a first step to using Twitter activity as one of the data sources for train prediction, we start with a simple question: How do Twitter users currently feel about Caltrain?
For this month’s Throwback Thursday, a post that provides insight and concrete advice on how to tackle imbalanced data.
In this interview, Paco Nathan discusses making life more livable, AI fears, and more.
Business leaders cannot afford to ignore their organization’s data—rather, that data should be used to make informed decisions. In this post, Principal Data Scientist Tom Fawcett and Professor of Data […]
This past August was the first JupyterCon—an O’Reilly-sponsored conference around the Jupyter ecosystem, held in NYC. In this post we look at the major themes from the conference, and some top talks from each theme.
You should understand whether the right things have been measured and whether the results are suitable for the business problem.
We (Tom, a Machine Learning practitioner, and Drew, a professional Statistician) have worked together for several years. We believe we have an understanding of the role of each field within data science, which we attempt to articulate here.
The Data Strategy track of our webinar series focuses on creating and continuously updating your data strategy. Register now!