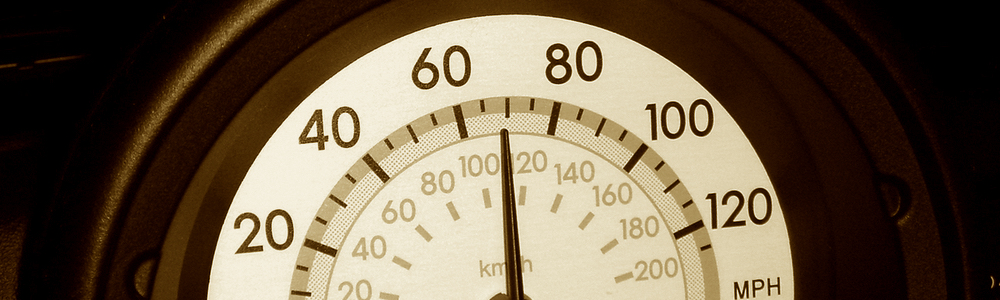
Getting Value Faster with a Data Strategy
December 15th, 2015
There’s a running joke in our industry about calls to IT service companies from client executive teams who “want to install some Hadoops.” The joke stems from the many businesses, eager to get in on the advantages of using data, who want to quickly install some software and get magic results. If you work with data then you know that these “Hadoops” (or any other tool or platform) can’t magically address underlying business concerns.
A McKinsey-Oxford survey discovered that, of the major IT projects that had significant cost overruns, the largest contributing factor was unclear objectives and a lack of business focus. If you don’t take the time to carefully define the set of problems you need to solve up front, then you’ll either be left with a system that was designed for only a narrow sliver of use cases, or one that becomes bloated with add-ons meant to address a multitude of issues in whack-a-mole style. Either way, the resulting system will probably be abandoned shortly after the initial project ends.
Companies that demand immediate technical results often don’t want to take the time to develop a data strategy up front, but it will actually accelerate business value for your company. It will ensure that each of your technical build-outs directly address your most critical business goals.
You can find the significant details of a data strategy in our position paper, but as a quick overview, the process of creating a data strategy consists of the following components:
- Identifying your business goals and current capabilities
- Determining future requirements
- Designing a project roadmap prioritized to deliver value early
- Aligning business and technology stakeholders around technology investment
In this post, I’ll describe how these components fit together and work to bring you business value quickly.
Identifying your Goals and Capabilities
Pinpointing your priorities
Many of our prospective clients have related a similar story: a team at a product company hears about a new customer intelligence platform that will yield new capabilities for integrating and analyzing consumer data. It will provide a holistic customer view, predictive analytics, campaign analytics, and more. Unsurprisingly, the team jumps at the opportunity and kicks off an IT project to install or build the tool, despite the substantial effort involved.
At first, everyone seems to win. The company checks a box on IT investment, and the business analysts can now get a better view of their customer ecosystem. However, difficulties quickly pop up. The tool doesn’t work as advertised, for example, or it doesn’t produce results as quickly as the company had hoped. Perhaps the analysts need to create or modify models, but the tool will not easily allow it. Many times, the company has forgotten to ask key questions regarding the purchase in the first place, such as: What exactly do I want to learn about my customers? Do I have the right customer data to begin with?
We’ve encountered many situations in which no one asked such questions. Yes, the system helped the product company to answer a lot of questions about its customers, but those questions didn’t match the company’s key needs and priorities. We’ve seen many businesses with their needs unmet, despite their having purchased promising technical solutions.
When your strategy begins with your business priorities, you will ensure that you are solving your business’ most important problems instead of just picking one to address at random. A good data strategy helps you gain the biggest return by forcing you to address the items that will produce the largest impact first. This gives you the chance to plan ahead and identify which company groups and functions will be impacted by any new tools, and it allows you to adjust your strategy accordingly.
Devising a data strategy takes time, but a focused data strategy effort should take only 6–8 weeks. Yes, that is time in which you could be kicking off an engineering project, but at the end of that investment you will know which project makes the most sense as a starting point and how it fits into your overall roadmap. In the end, there is no wasted time and you’ve minimized a common risk for many large platform-led projects: surprises.
Cataloging your capabilities
After you prioritize your business goals, the next step is to identify the functional and technical capabilities required to meet those goals, along with the high-level use cases that bring those capabilities to life. This process has several advantages over simply building or installing a technical system right away. First and foremost, once you explicitly lay it out, you will know what specific capabilities you need to meet your goals—and those may not necessarily include the latest and greatest big data tool.
An important part of strategy assessment is also discussing these capabilities with project stakeholders (more on stakeholders later). This gives you the chance to verify whether multiple internal groups would benefit from the same capabilities, and whether those capabilities already exist somewhere in your company. You can then take those teams’ technical learnings into account when building out your future project roadmaps.
When identifying your desired functional and technical capabilities, a data strategy also gives you a comprehensive look into your data ecosystem. You have the opportunity to identify any data gaps up front. A goal may be to analyze the effectiveness of your consumer marketing campaign, but if you haven’t been capturing the proper data from your customer interactions, then even developing the best analytical models will not give you the results you need. Going through a data strategy assessment will keep you from losing valuable time scrambling to acquire necessary data after your new technical capabilities are already built.
Identifying Dependencies: Doing the foundational projects first
The next part (and benefit) of the data strategy process is mapping dependencies between priorities, capabilities, and projects. With these dependency mappings, you make sure you are solving your prioritized problems in the optimal order. For example, consider a scenario in which you’ve listed your business priorities, along with the technical capabilities needed to achieve them. It’s clear that you need a new customer analytics platform, and there are many small projects involved. Your predictive modeling project and customer data integration project are of equal priority, but for your purposes, the data integration will enable many more additional projects and capabilities than the predictive analytics. Thus, you can easily see that you should tackle the data integration project first.
Similar to how product startups focus on the minimum viable product (MVP), imagine a minimum viable capability (MVC). Mapping all of the dependencies while building the roadmap allows you to produce an MVC and then build upon that. Then you can combine dependent projects to produce results even faster. Through each subsequent project, the existing MVC is utilized and expanded to incrementally create your complete list of capabilities. As a result, you get to test your hypotheses early, measure results, and tweak as necessary.
For instance, instead of fully installing a large system before seeing any benefit, you can use a data strategy to plan out how to build smaller sets of functionality as they are needed. As an example, when integrating a subset of customer data, you can perform predictive modeling on that subset, then scale out the modeling as you integrate additional consumer data. As a result of these incremental projects, you end up seeing ROI and business results fairly quickly and can adjust your future strategy as necessary during each iteration. There is a small delay in that you haven’t built a technical system right away, but planning for these dependencies and the corresponding small, incremental projects assists you in achieving your desired results much more quickly. As an added note, these small IT projects are also seven times more likely to be successful (e.g., on-time and within budget) than larger, all-inclusive ones, according to a Standish Group research report.
Involving the Right Stakeholders
In addition to the above benefits, one final point to note is that creating a data strategy involves bringing together many different business and technical stakeholders from your organization. When you do so, you obtain everyone’s input at the beginning, instead of getting critical feedback after it’s too late.
Too often, data and analytics projects get kicked off without involving all of the affected parties. For example, the marketing analytics department plans and scopes a campaign analysis initiative, but they don’t involve the key IT or engineering stakeholders until later stages. This ultimately results in project delays, as the IT department may not have reserved infrastructure, allocated storage and processing, etc. Thinking through a data strategy with contributions from all relevant employees is key to identifying and avoiding problems early.
Roadmap: Tying it all together
After looking at the data, capabilities, use cases, and stakeholder concerns, the final, tangible deliverable from the data strategy process is a project roadmap directly based on your business objectives. Although there are many ways a roadmap can quickly deliver value for your company, the most significant is that it gives you real, meaningful use cases to tackle—instead of you simply picking an issue to go after without really looking at all your key business priorities holistically.
By giving you a comprehensive look at your business priorities, technical ecosystem, and stakeholder inputs first, a data strategy will help you avoid big problems later. Ultimately, this leads to faster value.