Previous Article
Wearable Medical Device — Customer Engagement
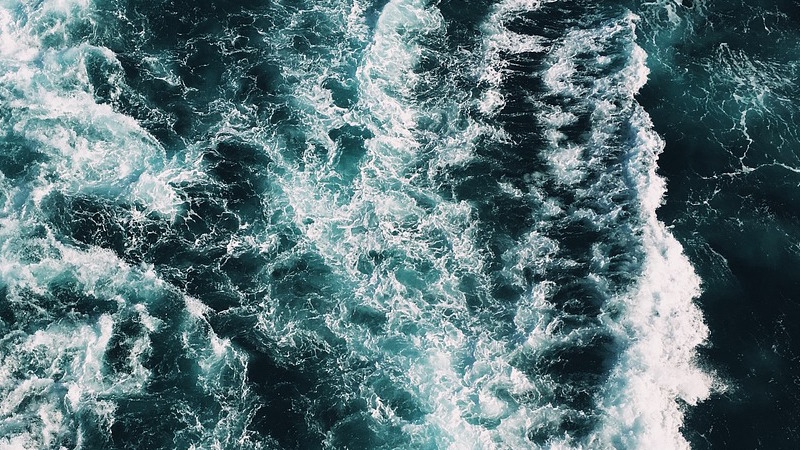
Summary
A leading wearable medical device company needed to understand why patients may discontinue treatment.
Silicon Valley Data Science helped the client predict who would churn, and how to prevent it.
Background and Business Problem
Our client provides a chronic disease monitoring solution. The organization speculated that a third of patients new to their device discontinued use in their first year, leading them to believe they had a very significant opportunity to improve patient engagement. Customer usage of the device is important both for business (so they repurchase disposables for the device) as well as helping patients better manage their condition. Understanding attrition is an important aspect of understanding patient engagement and the patient lifecycle, especially since customer acquisition is expensive to everyone involved—our client, health plans, and the patients themselves
Our client had difficulty defining and measuring patient churn, making it nearly impossible to intervene and address root causes. They needed to answer the following questions:
- Who has actually churned?
- Why did they churn and can we do anything about it?
- Can we predict who will churn before so that we can prioritize intervention efforts before it is too late?
Measuring churn is particularly challenging as the client’s sales are distributed across multiple channels that vary in breadth, availability, and quality of data. Furthermore, multiple different types of “signals” of churn exist across sales records, device and mobile app usage patterns, tech support, and even third party sources. Individually, none of the signals alone provides enough insight into the customer journey.
Solution
SVDS had previously worked with this client analyzing the data sources, proposing architecture, migrating candidate data into a new data platform, and connecting discrete elements of customer data. This engagement began with developing a data-driven definition of patient churn.
SVDS worked with business SMEs to clarify the following:
- What does it mean to “churn” and why is measuring it so hard?
- Who is interested in churn and why?
- What is the “prediction problem” we’re trying to solve?
- What are hypotheses about what could be causing churn?
Understanding, defining, and measuring churn is particularly challenging in industries like medical devices since products are sold through distributors, wholesalers, and pharmacies. Direct interaction with consumers is limited. To address this, we took time to evaluate which signals were patient generated and could be tied to device usage and engagement. This meant clearly defining what churn was measurable based on one specific channel. With that foundation, the models could be applied to learn about other channels.
It also meant defining patient usage patterns such as “one and done,” “active,” and “prescribed usage”; some customers would get the device, stock up on a three month supply, set up the app, and stop using it sometime before their replenishment order. Others would make conscious decisions to take breaks due to seasonality, changes in insurance coverages, or issues with the device. We had to classify the types of usage patterns and determine the likelihood of a customer re-ordering.
Given the variety of data, feature selection was critical. While there were dozens of features, honing in on the most relevant ones allowed for meaningful analysis to build prediction models and then test them against the population.
The specific project outcomes included:
- A metric that measures churn that was published in a company-wide dashboard, productionalized into customer-serving systems, and will eventually be communicated in external communications.
- A classifier that better identifies who specifically has churned in the past which, is critical to understanding drivers and creating training sets to predict future churn.
- Developing a predictive model that ranks likelihood of churn, which has been deployed to proactively intervene prior to patients discontinuing use of the device.
- Creating a reliable, repeatable measure of active patients.
- Through data, deriving drivers of churn based on product issues, cost, and customer behaviors.