Previous Article
Global Agriculture Company — Data Strategy
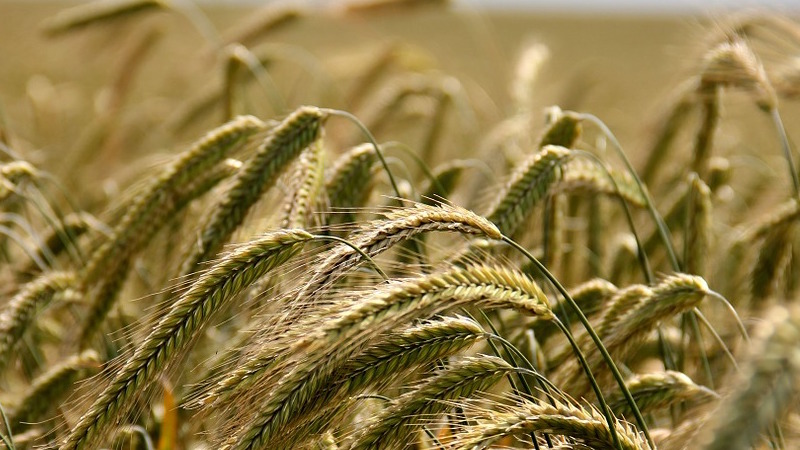
Summary
A global agricultural company wanted to grow its analytical capabilities to improve its decision making and provide digitally-integrated solutions to growers.
Silicon Valley Data Science provided a path forward to close gaps in their data assets and supporting analytics infrastructure.
Background and Business Problem
The new CIO at a global agriculture company had a globalization mandate and wanted high-value IT (not high-throughput IT), in order to digitize and modernize the seed pipeline. In response, the VP of IT in Products and Engineering created centers of excellence around information strategy, data science strategy, application strategy, and a mandate for a more modern architecture platform supporting these activities.
Along with a major organizational centralization of IT, our client embarked on this modernization path, built up teams of technical talent, migrated to new distributed platform architectures and gave sophisticated tools to their data scientists to draw insights from their various data assets. However, they struggled with several issues:
- Multiple analytics teams were using different approaches, data, and definitions for things that should have been standardardized across the company.
- Lack of coordination or centralization resulted in redundant efforts across teams.
- There were varying degrees of sophistication, as well as adoption rates, in how teams actually used the new platform.
- Disparate sources of data, and significant data siloes, prevented integration of strategic data assets across the company.
Our client wanted an independent validation of their data and architecture strategies with expert, prescriptive recommendations on how to accelerate progress and change direction, if needed. They also wanted recommendations to ensure the success of a specific broad strategic business initiative that was a first of its kind in connecting all major divisions. The outcomes of the assessment would enable a holistic and systematic approach to deploying and realizing the benefits of their digitization efforts directed at both internal decision making and external grower outcomes.
Solution
SVDS conducted interviews of key stakeholders across all the major divisions to be able to understand their business objectives related to the strategic initiative. These were then translated into technical requirements and the underpinning data and analytics needs that would be necessary for success. We also conducted detailed interviews of the data science and technology groups as part of our extensive review of the client’s current state. We probed their architecture, platform design, components, data assets, related work flows of data science teams, and the points of friction being experienced along the data value chain.
We then leveraged our team’s subject matter expertise in the domain as well as technical knowledge to critically evaluate our client’s architecture, data gaps, and data maturity levels with respect to people, process, and system dimensions critical for success. In particular, they had critical needs to accelerate data science and engineering to improve scalability and platform responsiveness. We validated their current architecture and identified both strengths and obstacles that were causing significant friction for the teams. We also performed an evaluation of their key data assets and highlighted gaps in data performance that would impair their business goals. To further support calls to action, we mapped their technical workloads to their business objectives to inform technology investments based on business priority.
Finally, our maturity assessment revealed a need to:
- ensure clearly stated business goals are driving the analytic and technology investments, with an eye on demonstrative value and return on effort
- strengthen their analytical team and improve cross-team partnerships for better collaboration and handoffs between divisions
- create stable and repeatable processes to manage data and data pipelines to realize value from data assets
We concluded our assessment with specific calls to action to ensure the initiative’s success.
There were several areas of impact from this effort for our client. Their data stewardship team made the decision to adopt on a wholesale basis our data gap assessment methodology for all their other initiatives. They also responded to our recommendations and realized the benefits of closer collaboration between their data scientists and engineers which accelerated their analytic efforts. There was also general agreement on the architectural approaches to enhance their data value chain, which yielded significant improvements in adoption and ease of use.