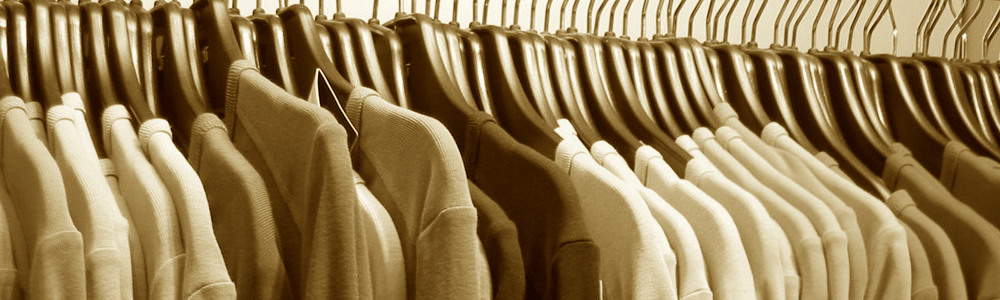
Driving the Digital Transformation in Retail and Hospitality
December 1st, 2015
A version of this post originally appeared on LinkedIn. Juergen will be speaking on this topic on December 2nd at the Chief Technology Officer Summit in San Francisco.
Shopping mall, retail, and hospitality companies in the United States and Western Europe are waging a war on several fronts. In the hospitality industry, profits are under threat from the sharing economy players such as AirBnB, Homeaway, and VRBO. Retailers too are competing against focused online players who enjoy much better capital asset productivity and who are now gaining ground even in the food category.
Established players have responded to these challenges posed by “digital native” players by launching a digital transformation of their own. They are leveraging the power of digital marketing, mobile devices, cloud, and big data to transform the customer experience and improve profitability.
This article highlights 12 initiatives centered on big data and analytics, which leading shopping mall, retail, and hospitality companies are pursuing as part of their digital transformation. These initiatives share four common characteristics:
- They create great moments for everyone every day. Analytics provide the insights that power a personalized customer experience. Digital channels — for instance smartphone applications or an e-commerce site — deliver that personalized customer experience in a seamless, frictionless way. Customers experience this in two ways: more “unique great moments” and fewer “wasted moments” (time spent waiting, searching, etc.)
- They create “stickiness” across the entire customer life cycle. They provide “pre-cash register” visibility by looking beyond transactions to consider every available interaction and observation. A holistic approach examines entire customer journeys across all touchpoints, channels, time periods, needs, and preferences. That approach sees customers in the context of their social circles. Marketing plays a pivotal role in this as the steward of the customer “golden record,” a 360º view of the customer that is the foundation for highly targeted segmentation and personalized calls to action.
- They treat data as a production factor similar to talent and capital. Joining and correlating data sources across many different domains creates economic value. The technical foundation for many of these initiatives is an enterprise-wide pool of data — commonly referred to as a data lake — that allows to acquire all data sources in their original format and store that data in one place, cost effectively and for a practically unlimited time. Such data lakes have been deployed by many leading retailers in the US already, promising better insights into potentially all aspects of a retailer’s business through the use of more powerful analytical processing engines. Existing business intelligence tools — notably enterprise data warehouses and relational databases — are not well suited for the volume, velocity, and variety of data sources found in a digital transformation. Traditional business intelligence systems holding ERP, CRM, and POS data are therefore extended with clickstream data, sentiment data, location data, and sensor data.
- They ensure successful outcomes by running big data initiatives as business transformations. In our experience, 50% of enterprises that embrace big data struggle with the complexity and skills required. Only 10% become truly successful. Those companies that truly succeed run big data initiatives as end-to-end cross-functional transformations of the business, not as mere technology projects. They are using the agile approach to software development that as perfected in Silicon Valley to design innovative big data systems and build data-driven solutions. And they take a realistic look at the competencies required for success.
The following big data and analytics initiatives are often deployed together with social and online marketing, mobile applications, and e-commerce in what is called the SMAC technology stack of digital transformation.
Customer experience use cases in retail
- In-mall experience. Analytics helps surface and remove friction from the physical shopping experience, notably by cutting down on wasted moments spent waiting or searching. For instance, visiting a large shopping mall during the weekend becomes “quite an expedition” as consumers navigate traffic jams on access roads, compete over scarce parking spots, and chart a path among hundreds of stores that satisfies everyone in the family. Location-based services (GPS tracking), sensors that detect flows of humans and cars, as well as the ubiquitous cameras already installed in malls jointly form a real-time heat-map that intelligently routes vehicle traffic and allows consumers to navigate the many retail experiences on offer more efficiently. A mobile application allows to reserve a parking spot and summon a concierge to carry the bags. In the future, it might even recall a self-driving car from its parking spot, analogous to the service that is already being provided by human valets today summoned via the Luxe app in San Francisco and other leading cities.
- In-store experience. Analytics in conjunction with a mobile app can also vastly improve the in-store experience. Walmart, for instance, developed a mobile app that switches to in-store mode once consumers approach the physical store. In mid-2015, 55% of consumers’ total online interaction with Walmart was via the mobile app. One of the most popular features is called PriceCatcher, which automatically matches the best price on offer by competitors. This not only underlines Walmart’s value proposition of “everyday low prices,” but it also improves loyalty of the most price-sensitive segment of shoppers. Other features include in-store navigation, personalized offers, shared shopping lists similar to the Wunderlist app, and faster check-out as pre-ordered items (for instance from the pharmacy) are readied for pick-up. For the retailer, the mobile app provides the holy grail of “pre-cash register” visibility. The information from the app (including location and browsing behavior) is complemented via information from in-store sensors, RFID tags, QR codes and iBeacons. The information is also used by Walmart to improve in-store layout, merchandising and product placement. The results are impressive: Walmart reports increased foot fall, larger basket sizes and a 40–70% customer lifetime value lift for those customers using the app.
- Cross-channel customer journeys. Analytics is also a key ingredient to achieving a seamless omni-channel experience. Customer journeys are increasingly starting online even for in store purchases. In some big-ticket categories such as electronics, 50–60% of shoppers are checking prices on their mobile phones. One way to offer a great moment for consumers who research online to purchase off-line is to offer click-and-collect capability. Another is to offer same-day delivery. Clearly many things need to fall in place to support this capability. Many retailers struggle with accurate and granular inventory information to support these use cases. Moreover, home delivery requires accurate address information, yet some fast growing markets such as the Middle East and China have not developed the street address system familiar in the US and Europe. Maps are quickly out of date given the rapid pace of urbanization. China has solved these logistics problems with geo-location data from consumer smart phones, which side-steps the street addressing issues. A surprising sight in many of the dense urban areas of China are bicycle rickshaws with better point-to-point geo-location and better GPS-enabled devices than you see at FedEx or UPS. Other examples of cross-channel customer journeys include show-rooming and social shopping. In a show-rooming scenario, consumers scan the barcode of an item in store with the intent of completing a transaction online, likely with a competitor. A mobile app can be used for re-targeting those consumers, for instance via a tailored offer. Social shopping is particularly popular among female apparel shoppers, and allows friends to weigh in (“look what I am getting”). Both of these scenarios are powered by big data & analytics, which provides fine-grained segmentation, personalization, search & discovery, and recommendation engine capabilities.
- Consumer finance, mobile payments and loyalty. Consumer finance and loyalty is not a new category. In the 1800s merchants on London’s Bond Street would typically extend 6–9 months credit to their customers. Loyal customers were likely to negotiate better prices, since fixed prices were virtually unknown and haggling was the order of the day. The modern-day store loyalty card dates back to the 1980s. What is new today is that retailers know a lot more about the life of their customers. Co-branded credit cards provide deep insights into consumer lifestyles and preferences, including a retailer’s likely share of wallet. Smartphone apps can generate targeted, personalized, real-time offers based on that rich context, for instance by matching customers’ locations to products and services that should appeal to them given their credit card purchasing habits and preferences. This can be further enriched by incorporating data from social networks (“likes,” “follows,” etc.). Marketing actions derived from this could include win-backs from competitors, efforts to increase basket size or to promote dining and entertainment in conjunction with shopping. Moreover, these personalized offers are linked directly to consumers’ loyalty cards or user accounts. One implementation of this is Apple Wallet/Apple Pay, which allows users to store coupons, event tickets, loyalty cards and credit cards on their smartphone.
Customer experience use cases in hospitality and entertainment
Hospitality and entertainment providers create great moments for everyone every day by recognizing customers across multiple channels and touchpoints to produce highly personalized experiences.
- Disney is using RFID wristbands which allow visitors to enter the park and purchase merchandise, enable guests to schedule reservations for rides, greet guests by name and function as hotel-room keys.
- Starwood allows consumers to personalize their experience via a mobile app. Guests can name their own check-in time for any trip through a feature called Your 24 Hours. They can check in to their room on-line on their way from the airport, and they can pre-order food to the room. Another hospitality company deployed a contact center solution called SATMAP, which pairs call center agents with callers based on personality matching, resulting in higher rates of customer satisfaction and sales conversion.
- Caesars has deployed a big-data driven application amongst its front-line staff to improve both process quality and customer experience. For instance, if a customer had a particularly unlucky day at the casino, front-line staff receive real-time alerts and have the authority to offer customers an upgrade or a free meal.
- A large hotel chain has deployed an app which reduces fraud in its restaurants. The company estimated that fraud is 4% of sales, as servers void or comp items after receiving cash payments from customers. This particularly impacts beverage sales, which “increased” by 10% following the introduction of the new application that allows restaurant managers to see where an employee is on a normal distribution. Food and beverage often accounts for as much revenue in a hotel as accommodations. Since beverages typically carry 60–90% margin, drinks can account for half of all restaurant profits.
Operational excellence use cases in retail
- Rent optimization. Big data provides mall operators with deeper insights that can be used to attract, serve, and retain tenants. Those insights might allow mall operators to extract higher rents or experiment with innovative retail concepts. For instance, insights on mall visitor traffic flows, dwell times, nationality, and other behavioral characteristics might be used to optimize the mix of base rent versus percentage rent. It might also help tenants improve their marketing activities, assortments or staffing.
- Price optimization. Big data provides much more dynamic price gathering and price optimization capabilities. A hypermarket featuring 20,000 to 40,000 items might have 1,000 so-called key value items, arranged in a few dozen key value categories. Some of these drive the perception of value (“good deals”) or the perception of assortment quality (a distinctive product selection). Others drive traffic (high-velocity items that inspire incremental shopping trips) or basket size. Traditionally, pricing for these key value items is relatively static. Big data automates a large number of manual price setting decisions. Moreover, pricing can be refined and managed in a much more agile fashion using new sources of insight and analytical capabilities.
- Inventory management. Big data helps reduce stock-outs and over-stocks by providing real-time, end-to-end visibility of product sales but also leading indicators of product demand in addition to deeper insights into the supply chain data across warehouses, drop ship vendors, and logistics partners. Text, click stream, social media, and sensor data complements more structured data on purchase history and product delivery times. This can be used to trigger automatic inventory replenishment.
- Vendor management. Category managers often struggle to analyze and rank the relative quality of their vendors across manifold KPIs such as vendor profitability, on-time service, customer feedback and complaints. An auction for logistics services in which drop ship vendors, third party logistics vendors, transportation vendors and packaging vendors participate illustrates the complexity. More than 100 suppliers bid for some or all of 10,000 discrete routes, each with more than 20 characteristics like “frequency” and “temperature control.” Traditional systems fail to take account of the complexity of unstructured text, image, and GPS data contained in bids.
- eCommerce. The leading eCommerce players such as Amazon pioneered the use of big data in retail. eBay co-invented Hadoop, a seminal big data technology. Well-documented big data use cases in eCommerce include product recommendation engines, web path optimization, and basket analysis.
Collectively, these digital transformation initiatives not only help create great moments for everyone every day, but they also have a direct impact on the key drivers of economic profit: revenue growth, margins, asset turns, and capital efficiency.