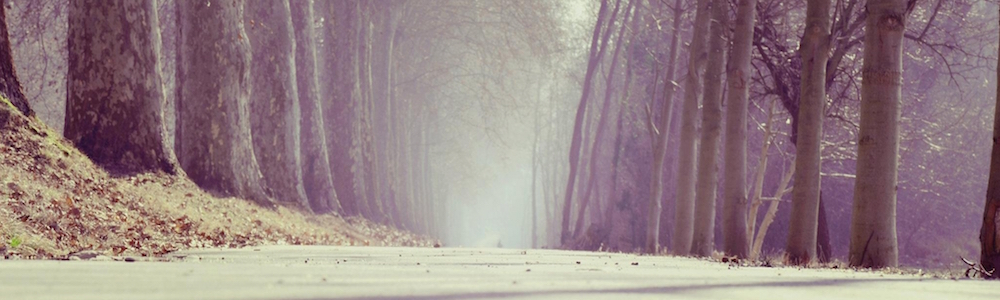
Data Strategy in a World of Big Data
October 25th, 2013
Silicon Valley Data Science has designed a new method to create a data strategy to overcome limitations of conventional approaches. Recent advances in communications, networking, data and analytics technologies are changing the way leading companies organize around, architect for, and deliver data-driven capabilities. That these organizations want to treat data as a strategic asset, rather than a cost center, has forced them to take fundamentally different strategies to unlock their data’s potential.
TRADITIONAL DATA STRATEGY
The early computer-based accounting systems for which conventional data management approaches were designed primarily reported financials to management and investors. These systems were designed to answer a set of known questions – i.e. “what was our company’s gross revenue in Wyoming in July 2012?” With a set of known, repeating questions to answer, data warehouse architectures and practices grew up to carefully curate the data inputs to the systems that answer those questions: Business Intelligence reporting systems.
Conventional approaches to data strategy can seem disjointed from the business needs of the enterprise beyond financial and operational reporting. These early data systems also existed in a data-poor, analog world. It is not surprising then, that legacy data management approaches begin from the perspective of the data and look to drive notions of quality – accuracy, completeness, timeliness, validity – without much thought for the uses to which the data may be put beyond the original reporting requirements for which the data was collected. There is an implicit assumption in these approaches that data of “high quality” is inherently valuable.
DATA VALUE AND QUALITY
There are two related dimensions of data that are critical to understanding data’s value to the enterprise: information value and quality. Notably, neither concept is particularly useful until you add “for what?”:
Information Value
There are several statistical methods to quantify and explore the information value of some independent variable (e.g. customer credit rating) with respect to a dependent variable (e.g. likelihood of receiving financing). However none of these techniques can be applied in the abstract, you can only use them if you know what target you are trying to understand the variance of. How can a company evaluate or quantify the value of some particular data, like customer credit rating, to the enterprise generally? There are no best practices or well-known heuristics.
Data Quality
Along similar lines, data quality in the abstract is difficult to pin down. Sensor data is notoriously noisy and “dirty”. Temperature sensors can drift over time. Such drift is important to correct for if the goal is to accurately measure the temperature of something. However, if there are methods of validating that reading, then maintaining the erroneous measurement enables analysis of sensor accuracy across vendors. One analysis needs the “cleansed” data, one needs the “raw” in the context of the “cleansed”, to be successful. Traditional approaches to data quality address data issues on write, and would typically not store the raw value.
Data strategies that seek to put governance, controls and process in place around data to drive abstract notions of quality in expectation of providing valuable information to the enterprise are bound to fail, or at the least struggle to be relevant, in modern digital enterprises.
A MODERN APPROACH TO DATA STRATEGY
A modern enterprise data strategy is critical in the digital age, where depth of operational sophistication and management of scale can be existential problems for growing enterprises. Fundamentally, data should serve the strategic imperatives of a business – those key strategic aspirations that define the future vision for an organization. An understanding of what business objectives – aspirational capabilities that an organization can take action to realize – are necessary to realize the strategic ambitions of a business is the foundation for building an enterprise data strategy. The data strategy itself is comprised of an integrated set of choices to support a drive to competitive differentiation.
For example, providing a differentiated customer experience is a strategic imperative for many businesses. Many such companies have a business objective of being able to provide customer-specific product recommendations. That actionable business objective then has its own associated data and technology needs that the data strategy should support. In this example customer and product information, and a recommendation engine are required to deliver customer-specific product recommendations.
SCALE-OUT ARCHITECTURES FOR DATA-DRIVEN ORGANIZATIONS
Continued successful product development requires a supporting infrastructure and architecture that can scale with the additional demands that come with experimentation and success. For data-driven companies, an infrastructure and data architecture that scales “out” rather than “up” is critical.
Scale-out architectures are a critical choice in a data-driven firm’s data strategy because they do not limit product development like scale-up architectures do. Scale-out’s defining characteristic is effectively stable (linear) incremental cost of data resource usage. Scaling with the experimental and production data requirements of a data-driven enterprise is only economical with scale-out architectures.
USE CASES & WORKLOADS
Data-driven companies invest in data architecture development, and data capability development to support their strategic imperatives. These investments should be prioritized primarily to deliver on the highest priority business objectives as early as possible. However, considerations such as dependencies, technical feasibility, availability of necessary skills, architectural suitability, and ease of production roll-out are important considerations as well.
The Silicon Valley Data Science approach to data strategy is to take that prioritized list of data workloads and build a roadmap of data capability and infrastructure development that builds sustainable advantage and superior value.
If you would like to connect your data strategy to your business objectives, contact us to find out how we can help!