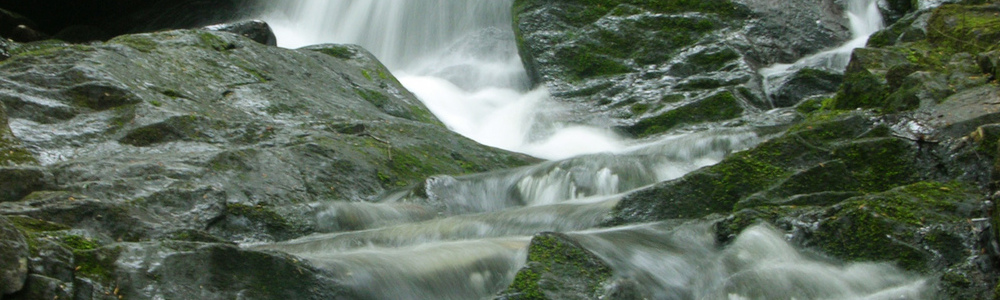
Structured Streaming in Spark
July 28th, 2016
Editor’s note: Andrew recently spoke at StampedeCon on this very topic. Find more information, and his slides, here.
Spark 2.0 (just released yesterday) has many new features—one of the most important being structured streaming. Structured streaming allows you to work with streams of data just like any other DataFrame. This has the potential to vastly simplify streaming application development, just like the transition from RDD’s to DataFrame’s did for batch. Code reuse between batch and streaming is also made possible since they use the same interface. Finally, since structured streaming uses the Catalyst SQL optimizer and other DataFrame optimizations like code generation, it has the potential to increase performance substantially.
In this post, I’ll look at how to get started with structured streaming, starting with the “word count” example.
Note: Structured streaming is still in alpha, please don’t use it in production yet.
Word Count
Word count is a popular first example from back in the Hadoop MapReduce days. Using a Spark DataFrame makes word count fairly trivial. With the added complexity of ordering by count this would look something like the code below.
Note that we are using the new SparkSession entry point (as spark) that is new in Spark 2.0, along with some functionality from Datasets.
val df = spark.read.text("/some/data/") val counts = (df.as[String] // Convert to Dataset[String] .flatMap(_.split(" ")) // Split into words .groupBy("value") // Group by word (column is "value") .count() // Count each group .orderBy($"count".desc)) // Sort by the count in descending order counts.write.csv(...)
If you wanted to accomplish the same thing in the old Spark streaming model you would be writing quite a bit of code because it relied on the older RDD interface and required you to manually track state. However, with structured streaming we can do the following.
val df = spark.readStream.text("/some/data/") val counts = (df.as[String] // Convert to Dataset[String] .flatMap(_.split(" ")) // Split into words .groupBy("value") // Group by word (column is "value") .count() // Count each group .orderBy($"count".desc)) // Sort by the count in descending order val query = (counts.writeStream .outputMode("complete") .format("console") .start())
As you can see, no change to the logic is required. What has changed is that we used readStream instead of read to get the input DataFrame, and writeStream instead of write to do output. Also, the output is a bit different; in the batch mode we can persist the output in a number of ways, such as csv like we used above. Since structured streaming is alpha it is still lacking in output options. Above, I’m using the console output option, which prints the result of each batch to stdout.
Window Changes
If you are familiar with traditional Spark streaming you may notice that the above example is lacking an explicit batch duration. In structured streaming the equivalent feature is a trigger. By default it will run batches as quickly as possible, starting the next batch as soon as more data is available and the previous batch is complete. You can also set a more traditional fixed batch interval for your trigger. In the future more flexible trigger options will be added.
A related consequence is that windows are no longer forced to be a multiple of the batch duration. Furthermore, windows needn’t be only on processing time anymore, we can rearrange events that may have been delayed or arrived out of order and window by event time. Suppose our input stream had a column event_time that we wanted to do windowed counts on. Then we could do something like the following to get counts of events in a 1 minute window:
df.groupBy(window($"event_time", "1 minute")).count()
Note that the window is just another column in the DataFrame and can be combined with other existing columns for more complex aggregations:
df.groupBy($"action",window($"event_time", "1 minute")).agg(max("age"), avg("value"))
You may rightly wonder how this is possible without having an ever-increasing number of aggregation buffers sticking around so that we can process arbitrarily late input. In the current alpha version there is no optimization for this. In the future, though, the concept of discard delay will be added, which will allow you to specify a threshold beyond which to discard late data so that aggregations can eventually be finalized and not tracked.
Sources
Currently, only the file based sources work. But there are some details you need to be aware of:
- File formats that normally infer schema (like csv and json) must have a schema specified.
- New files must be added to the input directory in an atomic fashion; usually this would be accomplished by moving the file into the directory after creation.
There is also a socket source which is only suitable for testing and demos, see the examples included in the release for usage. A Kafka source is also planned to be released shortly after the Spark 2.0 release.
Sinks
Before I discuss sinks, we first need to explore output modes. There are three modes: append, complete, and update. Append can only be used when there is no aggregation taking place. Complete outputs everything at the end of every batch. Update will do an update in place to a mutable store, such as a SQL database. Currently only append and complete are implemented.
The current options for sinks are extremely limited. Parquet output is supported in append mode, and there is also a foreach sink available for you to run your own custom code that works in append or complete modes. Additionally, there are console and memory sinks that are suitable for testing and demos.
Conclusion
Structured streaming is still pretty new, but this post should have given you an overview of why it’s an exciting feature. Have you tried it out at all? Let us know in the comments.